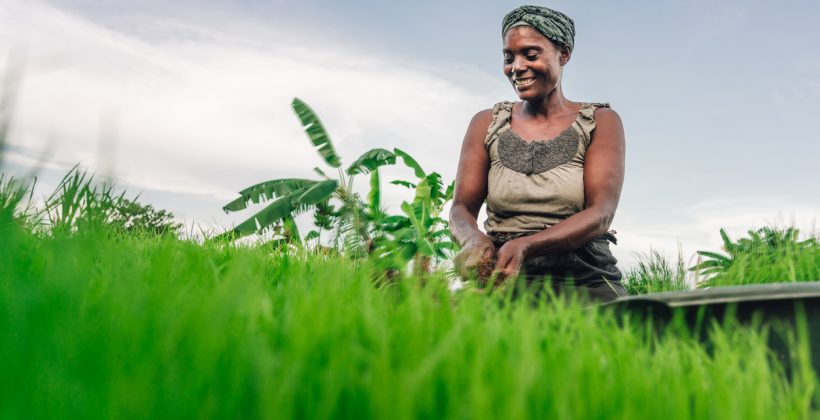
After launching its Young Africa Works strategy, the Foundation wanted to learn more about how their pre-Young Africa Works financial inclusion portfolio may (or may not) have led to employment creation for people on low-incomes, many of whom are unemployed or under-employed individuals working in agricultural production and agricultural supply chains.
However, most of their projects in the old financial inclusion portfolio were not designed to promote employment creation. Monitoring and evaluation systems and indicators were also not set up with this objective in mind.
We wanted to understand how the five projects in this portfolio we selected as case studies could lead to employment creation and how the data could be used to estimate it.
1. Speak to people to understand the data
Speaking to people working directly with the data will give you a better understanding of how rigorous the available data is and if there are any underlying assumptions.
These conversations also might reveal that there is more data available than initially thought. Some partners or programmes have multiple donors with different reporting needs so a whole new set of data could exist that you initially haven’t considered.
Implementing teams might also have additional insights on how the programme is creating change and for whom. This adds more detail and nuance to the data and helps you to think through creative ways to measure change (see next tip).
2. Be creative about indicators and set realistic expectations
Sometimes the data just won’t be there. Take stock of what you have, what you don’t have and what you can reasonably expect to collect from this point forward.
For example, we found that there was more data we could use to calculate direct jobs (mostly in enterprises) than indirect jobs (mostly farmers and producers) and even less data was reported on induced jobs, which limited what our research could say about the wider employment effects of these interventions.
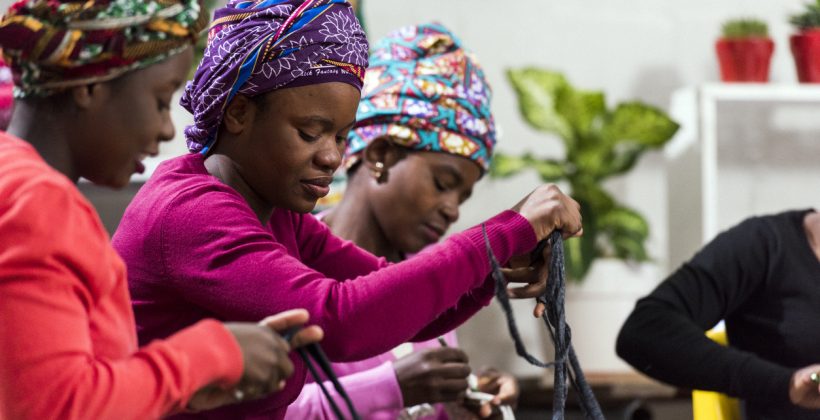
Where specific data isn’t available, you can consider using proxy indicators. In terms of rigour, the process of developing proxy indicators usually requires assumptions and the more assumptions you make, the more chance there is that one of those assumptions is not accurate.
This isn’t to say the process isn’t worthwhile – but it’s important to be transparent about the calculations and the limitations – especially if you want to compare results across programmes and if donors or implementers are using the indicators to inform decisions.
For the Mastercard Foundation, where jobs data wasn’t available, we used indicators related to income and agricultural production to understand the likely impact on employment creation (i.e. if production increases then it’s likely employment will increase among farmers and in the agricultural value chain).
3. Focus your efforts
Try to focus your efforts where you are most likely to find useful data. We adopted a case study-based approach, where our case studies were purposefully selected based on the availability of relevant data. We chose the case studies that could give us the greatest insight into the employment effects, the different measurement approaches and their relevance to the Foundation’s strategy.
4. Make sure you compare like with like
If you are comparing data across a range of projects, you can expect some inconsistency in how indicators are defined. Checking indicator definitions can help ensure you don’t start comparing (and aggregating) apples and oranges. It will also help you consider ways to convert data to draw more accurate comparisons.
Similarly to the use of proxy indicators (above), aggregating or comparing results often requires you to make some assumptions, so you need to be transparent about the calculations (and the limitations) and comfortable that the proxy indicators are credible (enough) to make comparisons.
For example, in our work with Mastercard Foundation, one partner had an indicator on ‘jobs supported’ which included the number of ‘farmers empowered’ and ‘artisans trained’.
Other partners reported on the number of individuals employed (without reference to their employment terms) and others reported on the number of full-time equivalent jobs. Where possible, we converted jobs data into full-time equivalent jobs.
Finding creative solutions
Overall, our key lesson is to take an approach that balances optimism with credibility. Too often M&E frameworks are seen as static and the quest for the perfect data becomes the enemy of ‘good enough’ data.
As MEL practitioners it’s our job to adapt alongside interventions and the information requirements of donors. This means being flexible and ready to present creative (and credible) solutions when learning and accountability objectives change.
If you’d like to learn more about this work, please contact Helen (helen.bailey@itad.com).